Providing health services to a widely-dispersed population can be a challenge. Here’s how AI can help when there aren’t enough medical professionals to go around.
China’s population, which in 2016 had 793 million urban residents and 590 rural residents, is spread out over a land mass of 3.7 million square miles.
By 2010, 93% of the rural population had healthcare coverage, but providing rural medicine and timely healthcare to rural regions persist. This is where analytics can make a difference.
“We wanted to take analytics, artificial intelligence and deep learning technologies and use them to better understand different medical conditions, how to diagnose them, and how to treat them,” said Kuan Chen, founder and CEO of Infervision, a Chinese artificial intelligence and deep learning company that specializes in medical image diagnosis.
Analytics, artificial intelligence, and deep learning are put into play by analyzing medical images and reports on different pathological conditions, and then coming up with different models and sources of treatment and medical interventions based on common patterns that are assembled from studies of thousands of patients in China’s urban hospitals. “These models use deep learning to ‘learn’ from the data and continuously improve their diagnostic capabilities,” said Chen.
The first disease that Chen targeted was lung cancer, with the software being able to locate hard-to-detect or hidden nodules in the lungs that could prove to be cancerous.
Now the task at hand is providing a similar diagnostic and medical intervention tool for strokes, which can especially be useful in rural areas where qualified medical practitioners are scarce.
How important is this?
“In many rural areas in China, there are no trained radiologists who can help stroke victims,” said Chen. “And in other areas of the world, like the US, radiologists make an average of $375,000 a year, so they are very expensive.”
Chen says that the feedback he gets from hospitals is that younger radiologists and medical practitioners rely heavily on AI, while older practitioners prefer to use it as a second opinion that they cross-check against their own.
“In a stroke, you want to respond to the condition as quickly as possible,” said Chen. “It might take 30 to 35 seconds in a standard process to generate a report on the condition so treatment can be determined. With our tool, that time is cut to less than three seconds.”
The use of deep learning and expanded analytics also expand the spectrum of diagnosis, which can lead to better results.
“In one non-stroke case that involved diagnosis and treatment of a bone fracture and a degenerated area of bone, the standard approach is to treat the affected area itself,” said Chen. “With analytics and AI, a system can focus on different areas of the body that are far removed from where the problem is to see if these other areas could be affecting the condition. If it is a problem that is being generated far from the fracture itself, the analytics allow us to treat causes of the condition, and not just symptoms.”
Here are some best practices hospitals and clinics can adopt as AI and deep learning tools evolve:
Deploy the tool where help is needed most
If there is an acute shortage of medical practitioners in a specific region, analytics and AI can help in situations like stroke intervention and treatment, and the chances for success for patients will improve.
Use the tool for training
Radiologists and medical practitioners must develop knowledge and experience before they can become expert diagnosticians. An analytics and deep learning tool can assist in the training process because users can compare their own findings against what the system finds in numerous scenarios.
Learn to expect the unexpected
You might think you are going to treat one condition and end up treating another. The bone fracture that Chen mentioned, where the system actually found the causal problem in a different area of the body, is a prime example. This is why medical practitioners should keep their minds open.
Never forget that AI and deep learning tools are still developing
Just because a system uses AI, deep learning and analytics doesn’t mean that is it always right. Medical practitioners should use these systems as assistants and not as undisputed authorities, because there are some areas where a machine can’t be a replacement for human thought and reasoning.
via: techrepublic

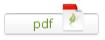
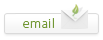

Leave a Reply